3. Non-Gaussian distributions
Non-Gaussian distributions, or probability distributions that are not Gaussian or normally distributed, present a challenge in Bayesian A/B testing due to the traditional Gaussian assumption often used in Bayesian inference.
Selecting an appropriate distribution to support accurate philippines girls telegram inference is difficult when the data does not follow a Gaussian distribution, which can lead to unreliable and invalid results from Bayesian A/B testing.
A common approach to address issues stemming from non-Gaussian distributions in Bayesian A/B testing is to transform the data to a Gaussian distribution, such as taking the logarithm of the data samples or through other suitable transformations.
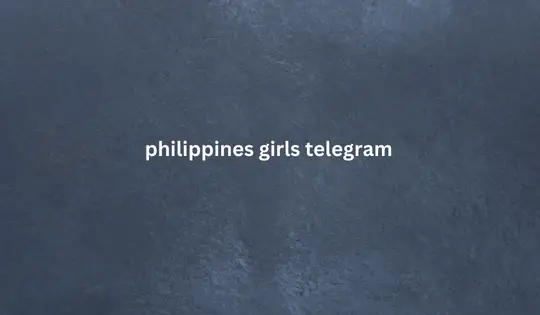
By doing so, traditional Gaussian-based Bayesian methods can be applied for analysis, ensuring accurate and reliable results in Bayesian A/B testing with non-Gaussian data.
Manual A/B testing, whether using the Bayesian or the Frequentist method, can be a real hassle.
OptiMonk’s Smart A/B Testing tool is a game-changer for businesses, offering fully automated testing for landing pages. With a “set it and forget it” approach, even marketers and business owners without CRO expertise can bid farewell to guesswork.
You have control over which elements you test, and you can effortlessly set up variants of your landing page. Simply launch your A/B test and let the AI do the heavy lifting, ensuring you get the best results without the need for constant monitoring or manual adjustments.